PyWAsP tutorial 4 PyWAsP - PyWake integration
Introduction
The example works through a complete wind turbine siting operation, starting with some measured wind data and ending up with a prediction of the power yield by erecting wind turbies at a specific site. This example includes AEP calculation which consider wake losses.
pywasp note: This is a classical step-by-step example from the
WAsP
help but extended with PyWake calculations of AEP.
Next, you will set up a wind farm consisting of several wind turbines and predict the annual energy production from this farm. Finally, we’ll map the wind resource over an area.
pywake note: To install pywake make sure you are in a conda enviroment containing
pywasp
and execute following command in a terminalpip install py_wake
pywake note: To install pywake PARK plugin make sure you are in a conda enviroment containing
pywasp
andpywask
and execute following command in a terminalpip install git+https://gitlab.windenergy.dtu.dk/TOPFARM/cuttingedge/pywake/pywake_park.git
The situation
The company Friends of Wind Energy, E-Corp Ltd. has asked you to provide a prediction of the power yield from locating a wind turbine in Serra Santa Luzia area in Portugal. They propose to erect a single 1-MW wind turbine at the summit of Serra Santa Luzia hill (they have modest energy requirements).
No wind measurements have been taken at the turbine site itself, but data have been collected from a meteorological station at nearby hill.
You are equipped with:
a contour map of the area
the wind data from the met station
a simple description of the land cover in the area
a description of the power-generating characteristics of the turbine
These data are located in the data
subfolder as following files:
a digital map of elevations and roughness
SerraSantaLuzia.map
a file containing wind data
SerraSantaLuzia.omwc
a data file containing a power production curve for the turbine
Bonus_1_MW.wtg
Working with WAsP to provide a prediction
From engineering data, you know how much power will be generated by the turbine at a given wind speed. If the plan was to erect the turbine at exactly the same place where the meteorological data had been collected, then it would be a really simple task to work out how much power to expect.
However, just from looking at the map it is obvious that the proposed turbine site is completely different from the meteorological station at the airport: the properties of the meteorological station itself will affect the wind data recorded there. In addition, the properties of the turbine site will have an effect on the way that the wind behaves near the turbine. It is also unlikely that the hub height of the turbine would be the same as the height of the anemometer.
What you need is a way to take the wind climate recorded at the meteorological station, and use it to predict the wind climate at the turbine site. That is what pywasp
does.
Using pywasp
, you can analyse the recorded wind data, correcting for the recording site effects to produce a site-independent characterization of the local wind climate. This site-independent characterization of the local wind climate is called a wind atlas data set or generalised wind climate. You can also use pywasp
to apply site effects to generalised wind climate data to produce a site-specific interpretation of the local wind climate.
Providing a prediction in the Waspdale case will therefore be a two-stage process (depicted in the right image). First, the data from the meteorological station need to be analysed to produce a wind atlas (“going up” in WAsP lingo), and then the resulting generalised wind climate needs to be applied to the proposed turbine site to estimate the wind power (“going down” in WAsP lingo).
Import packages
Usually the first step when writing a python program is importing standard and external packages we will need. For this analysis we will import numpy
, pandas
, matplotlib
, xarray
, pywasp
, pywake
and PARK pywake
plugin.
numpy
is python’s main numerical array package https://www.numpy.org/pandas
is python’s main package for manipulation of tabular data https://pandas.pydata.org/matplotlib
is python’s main plotting package https://matplotlib.org/xarray
is a powerful high level package for labelled multi-dimensional arrays http://xarray.pydata.orgpywasp
docummentation is found at http://docs.wasp.dk/py_wake
docummentation is found at https://topfarm.pages.windenergy.dtu.dk/PyWake/py_wake_park
docummentation is found at https://gitlab.windenergy.dtu.dk/TOPFARM/cuttingedge/pywake/pywake_park
As you work your way through the notebook, make sure to run the python code in each cell in the order that they appear. You run the code by clicking on the cell (outlines around the cell should appear) and pressing <shift> + <enter>
on the keyboard.
notebook note: if something looks wrong, or when errors occur, it can be helpful to restart the python kernel via the kernel tab in the top
[1]:
import warnings
warnings.filterwarnings("ignore") # We will ignore warnings to avoid cluttering the notebook
import numpy as np
import pandas as pd
import matplotlib.pyplot as plt
import xarray as xr
import pyproj
%load_ext autoreload
%autoreload 2
import pywasp as pw
import windkit as wk
import py_wake
from pywasp_tutorial import plot_bwc;
It is common to make a short alias of the package when importing using the import - as -
syntax. The functions and classes of imported packages must be accessed through explicitly typing the package name or alias, e.g. np.cos(3.14)
will use the cosine function from numpy
.
Observed wind climate
Now we will import our (observed) binned wind climate from the mast located at hill nearby Serra Santa Luzia hill where the turbine is going to be installed. As mentioned earlier, the binned wind climates is stored in SerraSantaLuzia.omwc
in the data
folder. It represents the observed wind climate for the period
1997-2002 at 25.3 m above ground level.
pywasp note
pywasp
includes functionality to both read and write wind climate files in many data formats, including ascii (.tab
), xml (.owc
and.omwc
), and netCDF (.nc
).
The geospatial coordinate in the file is in the World Geodetic System 1984, (EPSG:4326). We will tell pywasp
this by explicitly passing a keyword argument srs=4326
to the open_bwc
.
[2]:
bwc = wk.read_bwc('./data/SerraSantaLuzia.omwc', crs=4326)
print(bwc)
<xarray.Dataset>
Dimensions: (point: 1, sector: 12, wsbin: 32)
Coordinates:
height (point) float64 25.3
south_north (point) float64 41.74
west_east (point) float64 -8.823
crs int8 0
* wsbin (wsbin) float64 0.5 1.5 2.5 3.5 4.5 ... 28.5 29.5 30.5 31.5
wsceil (wsbin) float64 1.0 2.0 3.0 4.0 5.0 ... 29.0 30.0 31.0 32.0
wsfloor (wsbin) float64 0.0 1.0 2.0 3.0 4.0 ... 28.0 29.0 30.0 31.0
* sector (sector) float64 0.0 30.0 60.0 90.0 ... 270.0 300.0 330.0
sector_ceil (sector) float64 15.0 45.0 75.0 105.0 ... 285.0 315.0 345.0
sector_floor (sector) float64 345.0 15.0 45.0 75.0 ... 255.0 285.0 315.0
Dimensions without coordinates: point
Data variables:
wdfreq (sector, point) float64 0.05314 0.03321 ... 0.1148 0.0707
wsfreq (wsbin, sector, point) float64 0.02601 0.04219 ... 0.0 0.0
Attributes:
Conventions: CF-1.8
history: 2024-06-05T12:32:31+00:00:\twindkit==0.7.1.dev52+gc85bf...
wasp_header: SerraSantaluzia
Package name: windkit
Package version: 0.7.1.dev52+gc85bfbd
Creation date: 2024-06-05T12:32:32+00:00
Object type: Binned Wind Climate
author: Bjarke Tobias Olsen
author_email: btol@dtu.dk
institution: DTU Wind Energy
Notice that the bwc
object is of type <xarray.Dataset>
and that it contains four kinds of data:
Dimensions: core named dimensions
Coordinates: coordinate values along dimensions
Data variables: named arrays with data along 0-N named dimensions
Attributes: additional meta data attached to the dataset
xarray note the primitive datatype and dimensions of each variable are also shown, along with a small sample of the data.
wsfreq
is a four-dimensionalfloat64
(double precision) variable along dimensions(wsbin, sector, height, point)
Xarray datasets wrap numpy arrays, annotating them with human-readable dimensions and coordinates, and allowing for easy subsetting, data manipulation, and plotting of the underlying data. An xarray.Dataset
object is a collection of data variables, while each varible itself has type xarray.DataArray
.
xarray note Use the
.values
object attribute to access the underlying numpy array
Beyond the wind speed and wind direction distributions, the wind climate contains information about the height of the measurements (height
) and the geospatial location (west_east
and south_north
), which in this case hold the location information in the projected coordinates of the EPSG:4326 projection (i.e., south_north
=latitude
and west_east
=longitude
). However, as our terrain and rougness data are in the UTM Zone 29 (EPSG:32629) we will convert and update
the mast coordinates. Once the coordiantes are converted we will store them in in variables loc_x
and loc_y
for later use.
[3]:
bwc = wk.spatial.reproject(bwc, "EPSG:32629")
loc_y = bwc.south_north
loc_x = bwc.west_east
The next step is to plot the wind rose and wind speed distributions in the binned wind climate. For convinience a plotting function plot_bwc
has been implemented in pywasp_tutorial
that will do this.
notebook note: you can view the documentation for a function in jupyter notebooks by placing a
?
in front of the function, and you can get the entire function by using??
.
[4]:
wk.plot.histogram_lines(bwc)
As expected the prevailing wind direction at the site is from west, thus the Atlantic ocean.
Topography data
Now that we have loaded and inspected the wind climate, the next step is to the same with the terrain and roughness data. These data are used to calculate site effects used to generalize the observed wind climate (“going up” in WAsP lingo) and to downscale to nearby locations (“going down”).
The site effects are:
Orographic speed-up (by sector)
Internal boundar layer speed-up (by sector)
Effective upstream surface roughness (by sector)
pywasp note WAsP includes site effects due to obstacles, but we will not consider those here
Convienietly the terrain and roughness data are provided as a single vector map SerraSantaLuzia.map
. To load data we will use pw.read_vectormap()
.
Loading terrain and roughness data
To differentiate between the terrain and roughness parts of the vector map we need to tell pw.read_vectormap()
which map type it is reading along with the EPSG code. To display loaded data we convert the vector data to raster data using method to_rastermap()
and passing resolution as the argument (res = 100 m). The location of the mast is shown with an “X”.
Creating topography map
The elevation and roughness maps are the two components needed to make a TopographyMap
object in pywasp
, which is used to calculate the terrain effects.
In PyWAsP roughness map’s are automatically converted to a land cover maps with land cover id’s instead of roughness values. The corresponding roughness values for each id are stored in a conversion table, which is returned alongside the land cover map.
[5]:
elev_map = wk.read_vector_map("data/SerraSantaLuzia.map", crs="EPSG:32629", map_type="elevation")
pw.vectormap_to_rastermap(elev_map, res = 100).plot(figsize=(14, 10))
plt.plot(bwc.west_east, bwc.south_north, marker="X", color="white", markersize=10)
landcover_map, landcover_table = wk.read_vector_map("data/SerraSantaLuzia.map", crs="EPSG:32629", map_type="roughness")
landcover_raster = pw.vectormap_to_rastermap(landcover_map, res = 100, lctable=landcover_table)
landcover_raster.plot(cmap="tab20", figsize=(14, 10))
plt.plot(bwc.west_east, bwc.south_north, marker="X", color="black", markersize=10)
topo_map = pw.wasp.TopographyMap(elev_map, landcover_map, landcover_table)
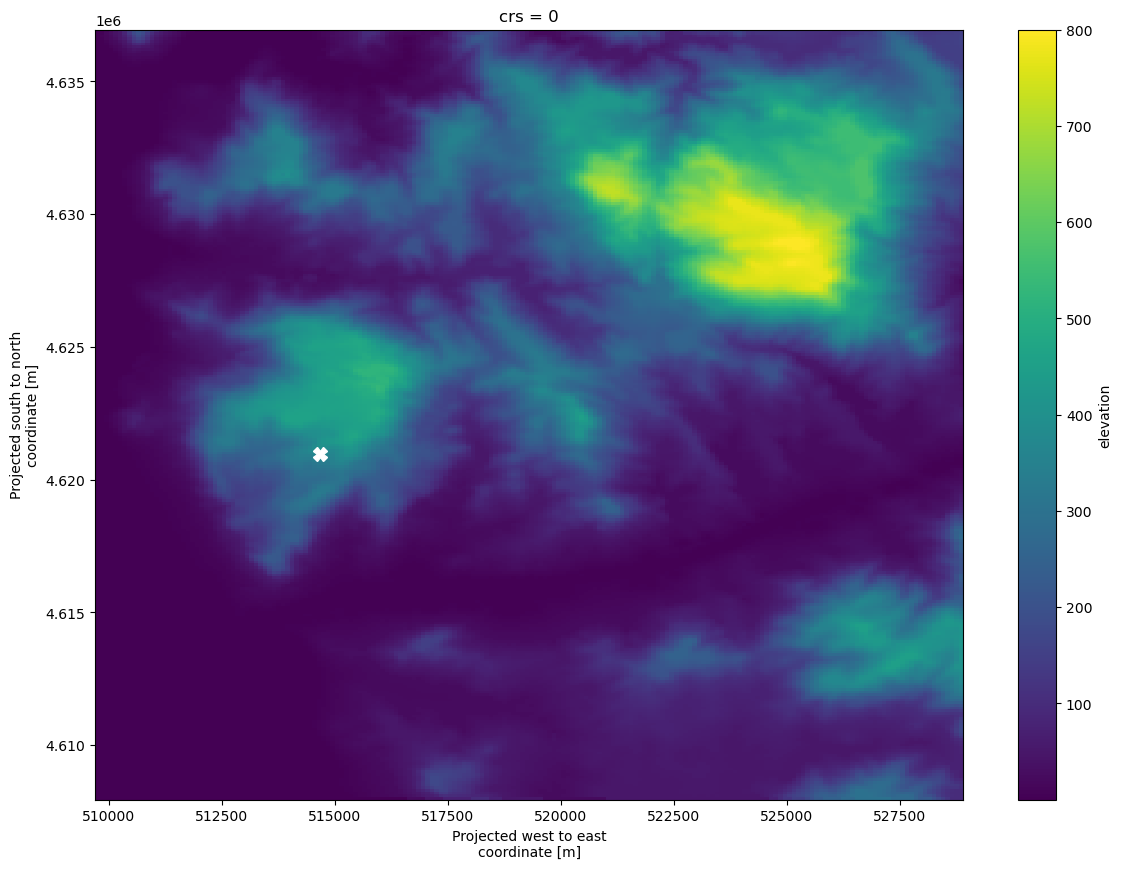
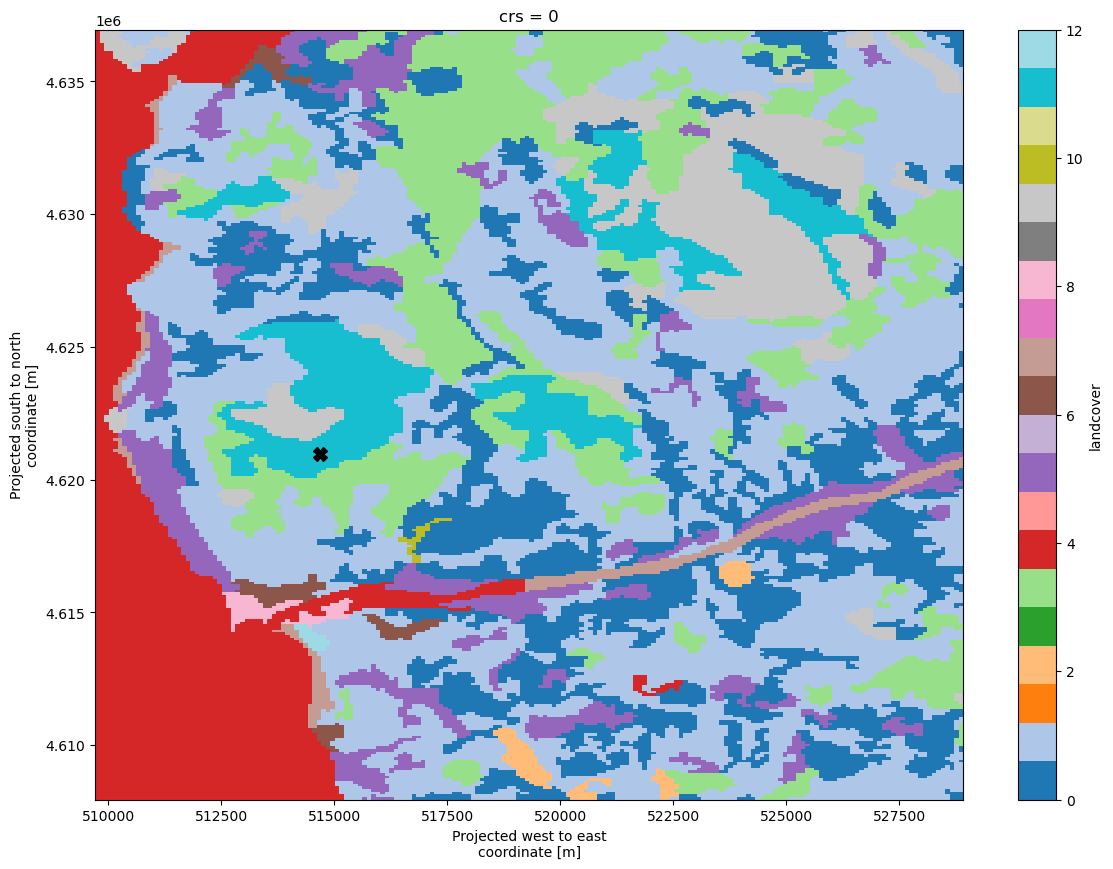
From the maps we can clearly observe the coast of the Atlantic ocean to the west as well the river Limia which dss into the ocean. Shown in red on the roughness map.
Output Locations
Next up we will take a look at the site effects for the future turbine location. We need to tell pywasp
what location we would like to calculate the site effects at. This is done by creating a xarray.Dataset
with dimensions (west_east
, south_north
, and height
) with coordinates values where we want to calculate the effects. Since this is a common need in PyWAsP, a convience function create_dataset
has been created for this purpose.
We tell pywasp
that the projection of west_east
, south_north
coordinates are in a UTM zone 29 by adding the attribute epsg
giving it the integer number 32629
.
[6]:
output_locs = wk.create_dataset(
np.array([514013.1]),
np.array([4617763]),
np.array([50]),
32629
)
print(output_locs)
<xarray.Dataset>
Dimensions: (point: 1)
Coordinates:
height (point) int64 50
south_north (point) int64 4617763
west_east (point) float64 5.14e+05
crs int8 0
Dimensions without coordinates: point
Data variables:
output (point) float64 0.0
Attributes:
Conventions: CF-1.8
history: 2024-06-05T12:32:35+00:00:\twindkit==0.7.1.dev52+gc85bfbd\t...
Site effects
Before generalizing the observed wind climate we read in earlier, we will take a look at the site effects at the mast location.
Let us first explore the roughness elements around the mast for each of 12 sectors. We can do this by using get_rou_rose
method of topo_map
, and pass in the location of the mast, note that roses are 2D so the height doesn’t matter in this case, and the number of sectors we would like to use. It requires a WAsP configuration object (pywasp.wasp.Config
) that stores the parameters. We will simply use the default values.
PyWAsP
will use the location information on the first object passed in (it must be a xr.Dataset
), so we can use the bwc
object to get the effects at the mast location.
[7]:
conf = pw.wasp.Config()
rou_rose, _ = topo_map.get_rou_rose(bwc, nsecs=12, conf=conf)
wk.plot.roughness_rose(rou_rose)
[8]:
conf = pw.wasp.Config()
output_locs_mast = wk.create_dataset(loc_x, loc_y, 0, 32629)
rou_rose, _ = topo_map.get_rou_rose(output_locs_mast, 12, conf)
wk.plot.roughness_rose(rou_rose)
The presence of the Atlantic ocean is clearly visible to the west, and we see that the rose corresponds well to what we would expect from the roughness elements we saw in the map.
To get the site effects we will use the get_site_effects
method from our topo_map
object.
get_site_effects
expects the unique west_east
and south_north
values, the number of sectors, and the height above ground, and the configuration object.
pywasp note depending on the number of locations,
get_site_effects
may take some time to complete
[9]:
site_effects = topo_map.get_site_effects(bwc, nsecs=12, conf=conf)
print(site_effects)
<xarray.Dataset>
Dimensions: (point: 1, sector: 12)
Coordinates:
height (point) float64 25.3
crs int8 0
west_east (point) float64 5.147e+05
south_north (point) float64 4.621e+06
* sector (sector) float64 0.0 30.0 60.0 ... 270.0 300.0 330.0
sector_ceil (sector) float64 15.0 45.0 75.0 ... 285.0 315.0 345.0
sector_floor (sector) float64 345.0 15.0 45.0 ... 255.0 285.0 315.0
Dimensions without coordinates: point
Data variables: (12/15)
z0meso (sector, point) float32 0.137 0.2776 ... 0.009736
slfmeso (sector, point) float32 0.9432 1.0 1.0 ... 0.403 0.5834
displ (sector, point) float32 0.0 0.0 0.0 0.0 ... 0.0 0.0 0.0
flow_sep_height (sector, point) float32 0.0 0.0 0.0 0.0 ... 0.0 0.0 0.0
user_def_speedups (sector, point) float32 1.0 1.0 1.0 1.0 ... 1.0 1.0 1.0
orographic_speedups (sector, point) float32 1.156 1.206 ... 1.43 1.284
... ...
orographic_turnings (sector, point) float32 -2.929 6.098 ... -4.751 -7.953
obstacle_turnings (sector, point) float32 0.0 0.0 0.0 0.0 ... 0.0 0.0 0.0
roughness_turnings (sector, point) float32 0.0 0.0 0.0 0.0 ... 0.0 0.0 0.0
site_elev (point) float32 381.0
rix (point) float32 0.04571
dirrix (sector, point) float32 0.001304 0.0005211 ... 0.06163
Attributes:
Conventions: CF-1.8
history: 2024-06-05T12:32:31+00:00:\twindkit==0.7.1.dev52+gc85bf...
wasp_header: SerraSantaluzia
Package name: windkit
Package version: 0.7.1.dev52+gc85bfbd
Creation date: 2024-06-05T12:32:37+00:00
Object type: Topographic effects
author: Bjarke Tobias Olsen
author_email: btol@dtu.dk
institution: DTU Wind Energy
title: WAsP site effects
The site effects dataset is made up of following variables:
z0meso
: effective upstream roughness by sectorslfmeso
: effective upstream sea-land-fraction by sectordispl
: displacement height by sectoruser_def_speedups
: user defined speed-ups (as factor)orographic_speedups
: speed-ups due to orography (as factor)obstacle_speedups
: obstacle induced speed-ups (as factor)roughness_speedups
: speed-ups due to roughness changes (as factor)user_def_turnings
: user defined wind turning (in degrees)orographic_turnings
: wind turning due to orography (in degrees)obstacle_turnings
: wind turning due to obstracels (in degrees)roughness_turnings
: wind turning due to roughness changes (in degrees)elev
: surface elevationrix
: ruggedness indexdirrix
: sector wise ruggedness index
The generalized wind climate calculation
Now it is time to actually generalize our observed wind climate and then downscale it to our chosen turbine location. The generalized wind climate is a site-independent characterization of the wind climate for the entire Waspdale area. Generalization involves calculating the wind speed for predefined surface roughnesses and heights above ground. So the first thing we need to do is chose these roughness and height classes. Typically, the wind climate contains up to 5 standard heights and 5 standard roughness classes.
In a generalized wind climate data set the wind observations have been ‘cleaned’ with respect to the site-specific conditions. The data sets are site-independent and the wind distributions have been referenced to five standard roughness classes and five standard height above ground level.
[10]:
gen_roughnesses = np.array([0.0, 0.001, 0.03, 0.1, 0.4, 1.5]) # 5 standard roughness classes
gen_heights = np.array([10.0, 50.0, 100.0, 150.0, 200.0]) # 5 standard height classes
The generalization is done with the generalize
function from pywasp
’s wasp
module. It takes the binned wind climate (bwc
), our topographic map (topo_map
), the roughness and height classes, and finally the configuration object (conf
).
[11]:
gwc = pw.wasp.generalize(bwc, topo_map, conf, gen_roughnesses=gen_roughnesses, gen_heights=gen_heights)
print(gwc)
<xarray.Dataset>
Dimensions: (point: 1, sector: 12, gen_roughness: 6, gen_height: 5)
Coordinates:
height (point) float64 25.3
south_north (point) float64 4.621e+06
west_east (point) float64 5.147e+05
crs int8 0
* sector (sector) float64 0.0 30.0 60.0 90.0 ... 270.0 300.0 330.0
sector_ceil (sector) float64 15.0 45.0 75.0 105.0 ... 285.0 315.0 345.0
sector_floor (sector) float64 345.0 15.0 45.0 75.0 ... 255.0 285.0 315.0
* gen_roughness (gen_roughness) float64 0.0 0.001 0.03 0.1 0.4 1.5
* gen_height (gen_height) float64 10.0 50.0 100.0 150.0 200.0
Dimensions without coordinates: point
Data variables:
A (sector, gen_height, gen_roughness, point) float32 5.69 .....
k (sector, gen_height, gen_roughness, point) float32 1.717 ....
wdfreq (sector, gen_height, gen_roughness, point) float32 0.06491...
site_elev (point) float32 381.0
Attributes:
Conventions: CF-1.8
history: 2024-06-05T12:32:31+00:00:\twindkit==0.7.1.dev52+gc85bf...
wasp_header: SerraSantaluzia
Package name: windkit
Package version: 0.7.1.dev52+gc85bfbd
Creation date: 2024-06-05T12:32:39+00:00
Object type: Geostrophic Wind Climate
author: Bjarke Tobias Olsen
author_email: btol@dtu.dk
institution: DTU Wind Energy
title: Generalized wind climate
The generalized wind climate contains weibull parameters (A
and k
) for each location, sector, and height and roughness class.
Estimating wind power
Now that we have calculated a generalised wind climate (GWC) with site-independent wind climate data, we can apply those data to prospect turbine sites. PyWAsP can adjust the data for the situation found at the turbine site and produce a prediction of the wind climate for the site itself.
The pywasp.wasp
module provides two ways of doing this: downscale
and get_wasp_down
. The latter assumes site effects that have already been calculated, while the first one will start by calculating them. Since we have already calculated the site effects, we will use get_wasp_down
. The function requires passing a genwc_interp
method. Two choices are available: 'nearest'
and 'natural'
. This is important when several generalized wind climates are present and will tell
pywasp
how to interpolate them to the location. Since just one wind climate is present in this study, the same generalized wind climate will be used everywhere.
To estimate the the power production for a turbine positioned at the site we need to add a turbine wind turbine generator object that holds the power curve and other information about the characteristics of the turbine. We will read a Wind Turbine Generator dataset using pw.io.read_wtg
. The turbine used here is the Bonus 1 MW wind turbine.
[12]:
wtg = wk.read_wtg("./data/Bonus_1_MW.wtg")
print(wtg)
<xarray.Dataset>
Dimensions: (mode: 1, wind_speed: 22)
Coordinates:
* wind_speed (wind_speed) float64 4.0 5.0 ... 24.0 25.0
* mode (mode) int64 0
Data variables:
power_output (mode, wind_speed) float64 2.41e+04 ... 1e+06
thrust_coefficient (mode, wind_speed) float64 0.915 ... 0.161
air_density (mode) float64 1.225
stationary_thrust_coefficient (mode) float64 0.161
wind_speed_cutin (mode) float64 4.0
wind_speed_cutout (mode) float64 25.0
rated_power (mode) float64 1e+06
name <U10 'Bonus 1 MW'
rotor_diameter float64 54.2
hub_height float64 50.0
regulation_type int64 2
Attributes:
Conventions: CF-1.8
Package name: windkit
Package version: 0.7.1.dev52+gc85bfbd
Creation date: 2024-06-05T12:32:39+00:00
author: Bjarke Tobias Olsen
author_email: btol@dtu.dk
institution: DTU Wind Energy
We can see that the dataset holds the information about the WTG, including: - Power and thrust coefficient curves for varying wind speeds - Wind speed cutin and cutout - Rated power - Rotor diameter - Hub height
The WTG can have different modes with different values for different air density situations for example. This WTG has 1 mode for the standard air density of 1.225 kg / m^3.
To plot the power and thrust coefficient curves we can use the plotting function plot_wtg_curves
from PyWAsP
:
[13]:
wtg["power_output"].attrs["long_name"] = "Power Output"
wtg["power_output"].attrs["units"] = "kW"
wtg["wind_speed"].attrs["long_name"] = "Wind Speed"
wtg["wind_speed"].attrs["units"] = "m/s"
wtg["thrust_coefficient"].attrs["long_name"] = "Ct"
wtg["thrust_coefficient"].attrs["units"] = ""
wk.plot.power_ct_curves(wtg)
Now that we have the turbine data we can combine it with a location in our map to predict the power. We will add the location and hub height of our turbine to xr.Dataset
we can create by using the built-in create_dataset
in PyWAsP
:
[14]:
x, y, hub_height = 514325, 4622375, 50.0
turbine_loc = wk.create_dataset([x], [y], [hub_height], crs="EPSG:32629")
Lets plot the turbine location on the elevation map to see where it’s located.
[15]:
fig, ax = plt.subplots(1, 1, figsize=(12, 8.5))
pw.vectormap_to_rastermap(elev_map, res = 100).plot(ax=ax, vmin=0, vmax=550)
ax.plot(turbine_loc.west_east, turbine_loc.south_north, marker="X", markersize=8, color="black")
ax.plot(bwc.west_east, bwc.south_north, marker="X", color="white", markersize=8)
ax.set(xlabel="west_east [m]", ylabel="south_north [m]");
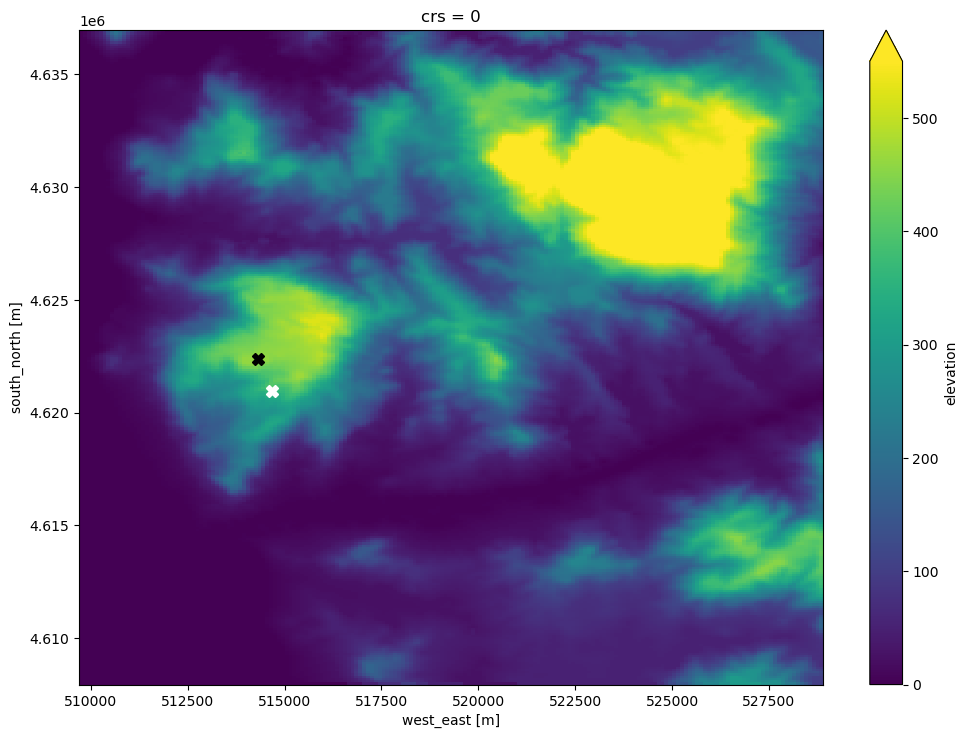
We can see that the wind turbine is located on a local peak on the hill to the west in the map near the coastline. Next up, we will calculate the power production for the turbine. To do this, we will first calculate the wind climate at the location of the turbine hub height using PyWAsP
’s downscale
function which takes in a generalized wind climate, the topography data, the turbine position(s) and hub height(s), the wasp configuration, and we also add the genwc_interp=nearest"
keyword to tell PyWAsP
we want it to use the nearest GWC to each turbine (in this case its always the same, since we only have one GWC).
[16]:
pwc = pw.wasp.downscale(gwc, topo_map, turbine_loc, conf, interp_method="nearest")
print(pwc)
<xarray.Dataset>
Dimensions: (sector: 12, point: 1)
Coordinates:
* sector (sector) float64 0.0 30.0 60.0 90.0 ... 270.0 300.0 330.0
height (point) float64 50.0
south_north (point) int64 4622375
west_east (point) int64 514325
crs int8 0
sector_ceil (sector) float64 15.0 45.0 75.0 105.0 ... 285.0 315.0 345.0
sector_floor (sector) float64 345.0 15.0 45.0 75.0 ... 255.0 285.0 315.0
Dimensions without coordinates: point
Data variables:
A (sector, point) float32 7.04 6.572 6.188 ... 7.326 7.172
k (sector, point) float32 2.041 2.385 2.498 ... 2.248 2.092
wdfreq (sector, point) float32 0.06301 0.0392 ... 0.09475 0.08239
site_elev (point) float32 460.0
air_density (point) float32 1.163
wspd (point) float32 6.998
power_density (point) float32 344.5
Attributes:
Conventions: CF-1.8
history: 2024-06-05T12:32:39+00:00:\twindkit==0.7.1.dev52+gc85bf...
title: WAsP site effects
Package name: windkit
Package version: 0.7.1.dev52+gc85bfbd
Creation date: 2024-06-05T12:32:42+00:00
Object type: Met fields
author: Bjarke Tobias Olsen
author_email: btol@dtu.dk
institution: DTU Wind Energy
We can now combine the predicted wind climate with the wind turbine generator to get the annual power production.
[17]:
aep = pw.wasp.gross_aep(pwc, wtg)
print(aep)
<xarray.Dataset>
Dimensions: (point: 1)
Coordinates:
height (point) float64 50.0
south_north (point) int64 4622375
west_east (point) int64 514325
crs int8 0
Dimensions without coordinates: point
Data variables:
gross_AEP (point) float32 2.568
Attributes:
Conventions: CF-1.8
history: 2024-06-05T12:32:39+00:00:\twindkit==0.7.1.dev52+gc85bf...
title: WAsP site effects
Package name: windkit
Package version: 0.7.1.dev52+gc85bfbd
Creation date: 2024-06-05T12:32:42+00:00
Object type: Anual Energy Production
author: Bjarke Tobias Olsen
author_email: btol@dtu.dk
institution: DTU Wind Energy
As we can see, the predicted power production for the turbine at the site is 2.6 GWh/y
Estimating wind farm production
Now that we have estimated the power for a single turbine, we can look at the power production for a whole wind farm. The workflow is indentical to the one we just used for a single turbine. We have predefined some turbine positions in the data/turbine_positions.csv
file, so lets create the location dataset and plot the turbines on our map.
[18]:
wtg_locs = pd.read_csv('./data/turbine_positions.csv')
output_locs = wk.create_dataset(wtg_locs.Easting.values,
wtg_locs.Northing.values,
wtg_locs['Hub height'].values,
crs="EPSG:32629")
fig, ax = plt.subplots(1, 1, figsize=(12, 8.5))
pw.vectormap_to_rastermap(elev_map, res = 100).plot(ax=ax, vmin=0, vmax=550)
ax.plot(output_locs.west_east, output_locs.south_north, ls="none", marker="X", markersize=8, color="black")
ax.plot(bwc.west_east, bwc.south_north, marker="X", color="white", markersize=8)
for i, (x, y) in enumerate(zip(output_locs.west_east.values, output_locs.south_north.values)):
ax.annotate(i, (x, y+50))
ax.set(xlabel="west_east [m]", ylabel="south_north [m]", xlim=(510000, 519000), ylim=(4620700, 4625500))
[18]:
[Text(0.5, 0, 'west_east [m]'),
Text(0, 0.5, 'south_north [m]'),
(510000.0, 519000.0),
(4620700.0, 4625500.0)]
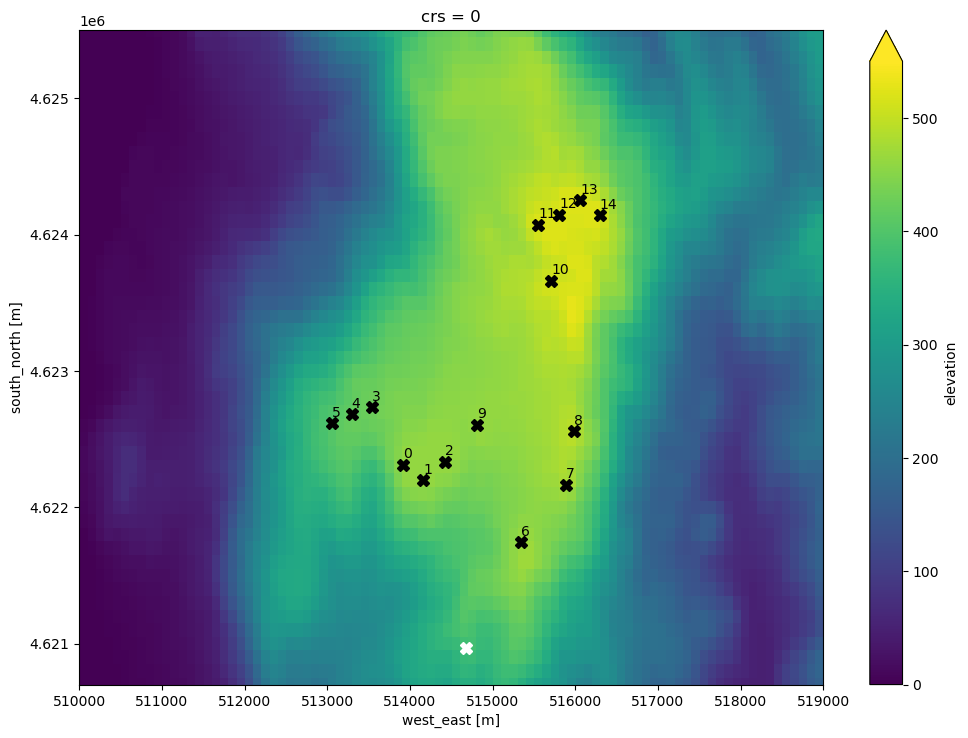
We can see that the turbines are mostly on the (locally) highest points in the terrain.
To calculate the predicted wind climates at the 15 positions (at 50 m AGL) we will again use the downscale
function.
[19]:
pwc = pw.wasp.downscale(gwc, topo_map, output_locs, conf, interp_method="nearest")
Now we can calculate the AEP
[20]:
aep_nowake = pw.wasp.gross_aep(pwc, wtg)["gross_AEP"]
print(aep_nowake)
print(aep_nowake.sum())
<xarray.DataArray 'gross_AEP' (point: 15)>
array([2.9344273, 2.794619 , 2.5766804, 2.6569452, 2.8252776, 2.9034677,
2.7838368, 3.0851603, 3.18083 , 2.4066768, 2.8184903, 3.058005 ,
3.0135605, 3.2057064, 3.4222102], dtype=float32)
Coordinates:
height (point) int64 50 50 50 50 50 50 50 50 50 50 50 50 50 50 50
south_north (point) int64 4622313 4622199 4622336 ... 4624252 4624142
west_east (point) int64 513914 514161 514425 ... 515808 516060 516295
crs int8 0
Dimensions without coordinates: point
Attributes:
description: Gross annual energy production, without considering wake ...
long_name: Gross annual energy production
standard_name: gross_aep
units: GWh
grid_mapping: crs
<xarray.DataArray 'gross_AEP' ()>
array(43.665897, dtype=float32)
Coordinates:
crs int8 0
We get back an AEP for each turbine. In total we estimate that our wind farm produces 43.7 GWh annually, without taking wake effects and other losses into account.
Lets now use wake effects in the AEP calculations to see how much these affect our annual power production. PyWAsP
uses PyWake
internally to take wake effects into account.
Several wake models can be used, but we will use the wake model that corresponds to the wake model found in the offical WAsP GUI software for Windows, called “PARK2” in PyWAsP
.
[21]:
aep_park2 = pw.wasp.potential_aep(
pwc[["A", "k", "wdfreq"]],
wtg,
wind_farm_model="PARK2_onshore",
turbulence_intensity=0.0
)["potential_AEP_sector"]
# Sum over sectors
aep_park2 = aep_park2.sum(dim="sector")
print(aep_park2)
print(aep_park2.sum())
<xarray.DataArray 'potential_AEP_sector' (point: 15)>
array([2.83374745, 2.70305958, 2.46916798, 2.54701922, 2.71523867,
2.86011705, 2.75593011, 3.03593558, 3.12073931, 2.33494791,
2.76085495, 2.97103928, 2.86495357, 3.03652726, 3.3034571 ])
Coordinates:
height (point) int64 50 50 50 50 50 50 50 50 50 50 50 50 50 50 50
south_north (point) int64 4622313 4622199 4622336 ... 4624252 4624142
west_east (point) int64 513914 514161 514425 ... 515808 516060 516295
crs int8 0
* point (point) int64 0 1 2 3 4 5 6 7 8 9 10 11 12 13 14
<xarray.DataArray 'potential_AEP_sector' ()>
array(42.31273502)
Coordinates:
crs int8 0
We can see that wake effects causes a reduction of the AEP from 43.7 to 42.3 GWh/y (a 3% reduction).
Let us visaulize the turbine-by-turbine AEP and reduction by wake effects.
[22]:
fig, axes = plt.subplots(1, 2, figsize=(15, 5))
aep_nowake.plot(ax=axes[0], marker="o", label=f"No wakes: {aep_nowake.sum().values:.1f} [GWh]")
aep_park2.plot(ax=axes[0], marker="o", label=f"PARK2 wakes: {aep_park2.sum().values:.1f} [GWh]")
(aep_park2/ aep_nowake).plot(ax=axes[1], marker="o", color="C1")
axes[0].legend(frameon=False, title="Wake model")
axes[0].set(ylabel="AEP [GWh]", ylim=(2.2, 4.4), title="")
axes[1].set(ylabel=r"AEP / AEP$_\mathrm{no wakes}$", title="");
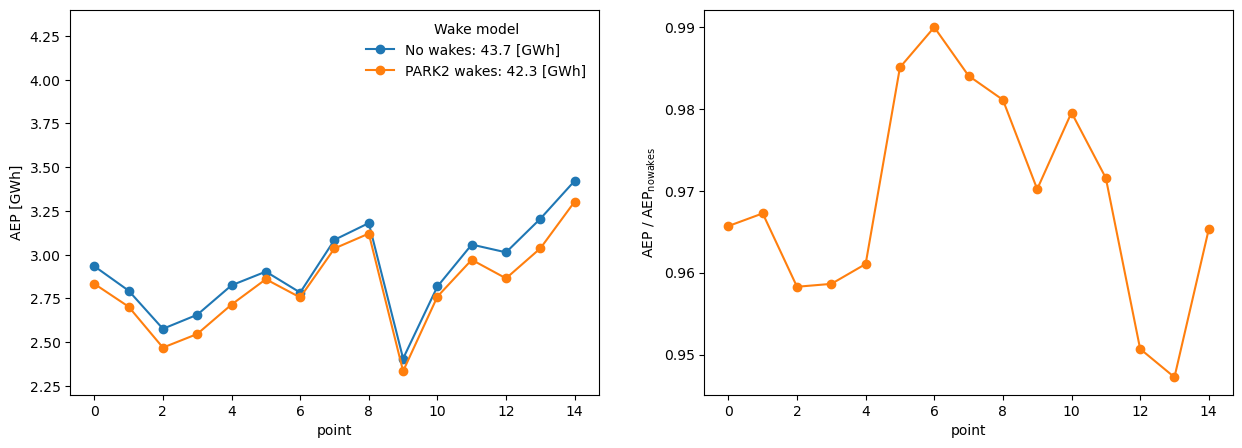
The First plot shows the AEP for each turbine. It varies alot from turbine to turbine due to the differences in the local wind climate.
The second plot shows the relative decrease in AEP due to wake effects. We can see that turbine at index 12 and 13 (Index starts at 0) are affected (relatively) most by wake effects, with their AEP reduced by about 5%.
[23]:
output_grid = wk.spatial.create_dataset(
np.linspace(511700.0, 517700.0, 41),
np.linspace(4619500.0, 4625500.0, 41),
[50.0],
crs="EPSG:32629",
struct="cuboid",
)
pwc_grid = pw.wasp.downscale(gwc, topo_map, output_grid, conf, interp_method="natural")
pwc_grid
[23]:
<xarray.Dataset> Dimensions: (height: 1, south_north: 41, west_east: 41, sector: 12) Coordinates: * height (height) float64 50.0 * south_north (south_north) float64 4.62e+06 4.62e+06 ... 4.626e+06 * west_east (west_east) float64 5.117e+05 5.118e+05 ... 5.177e+05 * sector (sector) float64 0.0 30.0 60.0 90.0 ... 270.0 300.0 330.0 crs int8 0 sector_ceil (sector) float64 15.0 45.0 75.0 105.0 ... 285.0 315.0 345.0 sector_floor (sector) float64 345.0 15.0 45.0 75.0 ... 255.0 285.0 315.0 Data variables: A (sector, height, south_north, west_east) float32 5.162 ...... k (sector, height, south_north, west_east) float32 1.982 ...... wdfreq (sector, height, south_north, west_east) float32 0.06678 .... site_elev (south_north, west_east) float32 15.3 22.06 ... 215.2 199.8 air_density (height, south_north, west_east) float32 1.216 ... 1.194 wspd (height, south_north, west_east) float32 5.545 5.387 ... 4.86 power_density (height, south_north, west_east) float32 200.1 ... 121.8 Attributes: Conventions: CF-1.8 history: 2024-06-05T12:32:58+00:00:\twindkit==0.7.1.dev52+gc85bf... Package name: windkit Package version: 0.7.1.dev52+gc85bfbd Creation date: 2024-06-05T12:33:05+00:00 Object type: Met fields author: Bjarke Tobias Olsen author_email: btol@dtu.dk institution: DTU Wind Energy title: WAsP site effects
[24]:
wtg_dict = {"bonus_1_mw": wtg}
wind_turbines = wk.create_wind_turbines_from_arrays(
west_east=output_locs.west_east.values,
south_north=output_locs.south_north.values,
height=output_locs.height.values,
turbine_ids=np.arange(len(output_locs.west_east.values), dtype=int),
group_ids=np.zeros_like(output_locs.west_east.values, dtype=int),
wtg_keys=["bonus_1_mw"]*len(output_locs.west_east.values),
crs="EPSG:32629",
)
flow_map = pw.wasp.wind_farm_flow_map(
pwc_grid,
wtg_dict,
wind_turbines,
output_grid,
wind_farm_model="PARK2_onshore",
turbulence_intensity=0.0,
n_subsector=5,
)
print(flow_map)
<xarray.Dataset>
Dimensions: (sector: 12, height: 1, south_north: 41,
west_east: 41, mode: 1)
Coordinates:
* sector (sector) float64 0.0 30.0 ... 300.0 330.0
* height (height) float64 50.0
* south_north (south_north) float64 4.62e+06 ... 4.626...
* west_east (west_east) float64 5.117e+05 ... 5.177e+05
crs int8 0
* mode (mode) int64 0
Data variables: (12/15)
potential_AEP_sector (height, south_north, west_east, sector) float64 ...
gross_AEP_sector (height, south_north, west_east, sector, mode) float64 ...
AEP_deficit_sector (height, south_north, west_east, sector, mode) float64 ...
wspd_sector (height, south_north, west_east, sector) float64 ...
wspd_eff_sector (height, south_north, west_east, sector) float64 ...
wspd_deficit_sector (height, south_north, west_east, sector) float64 ...
... ...
gross_AEP (height, south_north, west_east, mode) float64 ...
AEP_deficit (height, south_north, west_east, mode) float64 ...
wspd (height, south_north, west_east) float64 ...
wspd_eff (height, south_north, west_east) float64 ...
wspd_deficit (height, south_north, west_east) float64 ...
turbulence_intensity_eff (height, south_north, west_east) float64 ...
Attributes:
Conventions: CF-1.8
history: 2024-06-05T12:32:58+00:00:\twindkit==0.7.1.dev52+gc85bf...
Package name: windkit
Package version: 0.7.1.dev52+gc85bfbd
Creation date: 2024-06-05T12:33:19+00:00
Object type: Wind Farm Flow Map
author: Bjarke Tobias Olsen
author_email: btol@dtu.dk
institution: DTU Wind Energy
title: WAsP site effects
[25]:
fig, axes = plt.subplots(2, 3, figsize=(15, 7.5))
flow_map["wspd_sector"].sel(sector=240).plot(ax=axes[0, 0], levels=np.linspace(2.0, 10.0, 17))
flow_map["wspd_eff_sector"].sel(sector=240).plot(ax=axes[0, 1], levels=np.linspace(2.0, 10.0, 17))
(100.0 * flow_map["wspd_deficit_sector"]).sel(sector=240).plot(ax=axes[0, 2], levels=np.linspace(-10.0, 10.0, 21))
flow_map["wspd"].plot(ax=axes[1, 0], levels=np.linspace(2.0, 10.0, 17))
flow_map["wspd_eff"].plot(ax=axes[1, 1], levels=np.linspace(2.0, 10.0, 17))
(100.0 * flow_map["wspd_deficit"]).plot(ax=axes[1, 2], levels=np.linspace(-10.0, 10.0, 21))
for ax in axes.flat:
ax.plot(output_locs.west_east, output_locs.south_north, ls="none", marker="X", markersize=8, color="black")
ax.plot(bwc.west_east, bwc.south_north, marker="X", color="white", markersize=8)
ax.set(title="")
plt.tight_layout()
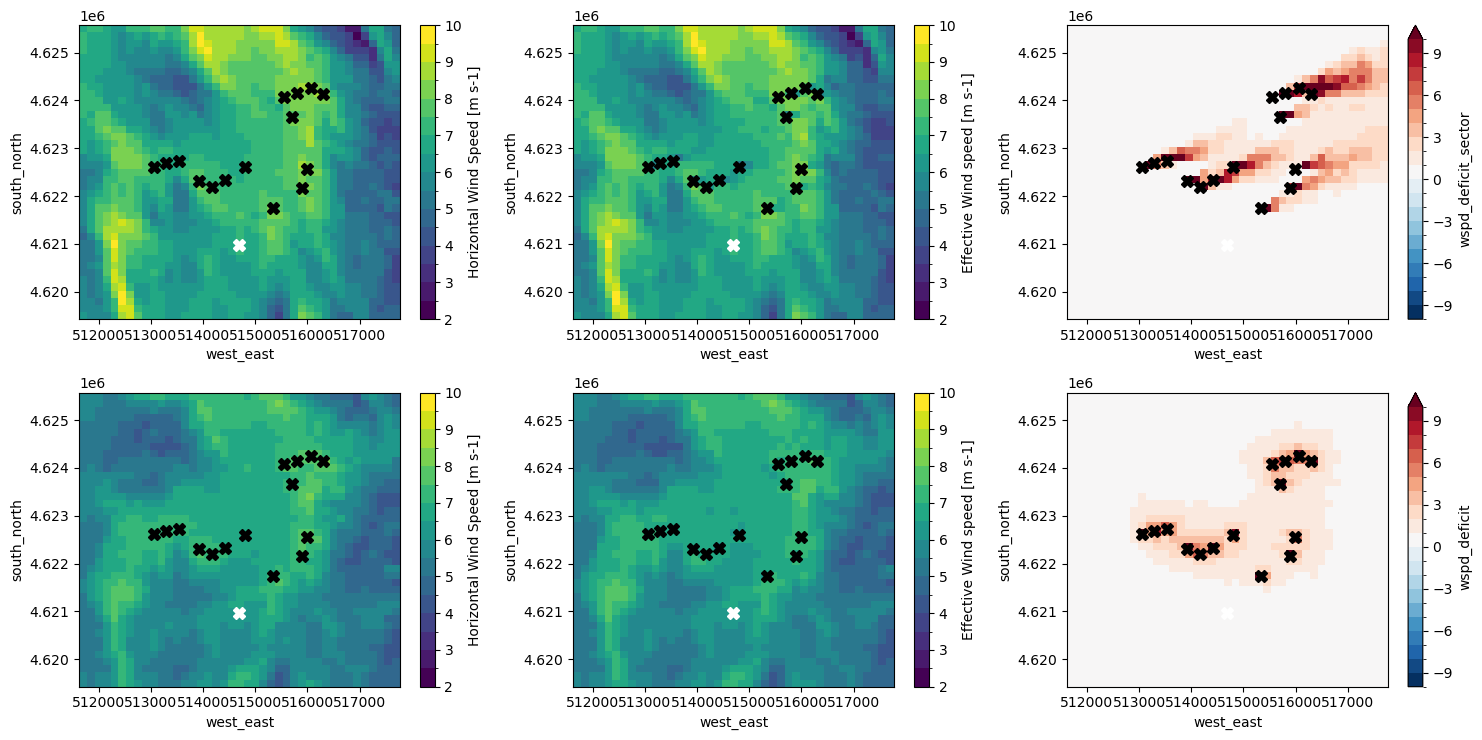
[26]:
fig, axes = plt.subplots(2, 3, figsize=(15, 7.5))
flow_map["gross_AEP_sector"].sel(sector=240).plot(ax=axes[0, 0])
flow_map["potential_AEP_sector"].sel(sector=240).plot(ax=axes[0, 1])
(100.0 * flow_map["AEP_deficit_sector"]).sel(sector=240).plot(ax=axes[0, 2], levels=np.linspace(-20.0, 20.0, 21))
flow_map["gross_AEP"].plot(ax=axes[1, 0])
flow_map["potential_AEP"].plot(ax=axes[1, 1])
(100.0 * flow_map["AEP_deficit"]).plot(ax=axes[1, 2], levels=np.linspace(-20.0, 20.0, 21))
for ax in axes.flat:
ax.plot(output_locs.west_east, output_locs.south_north, ls="none", marker="X", markersize=8, color="black")
ax.plot(bwc.west_east, bwc.south_north, marker="X", color="white", markersize=8)
ax.set(title="")
plt.tight_layout()
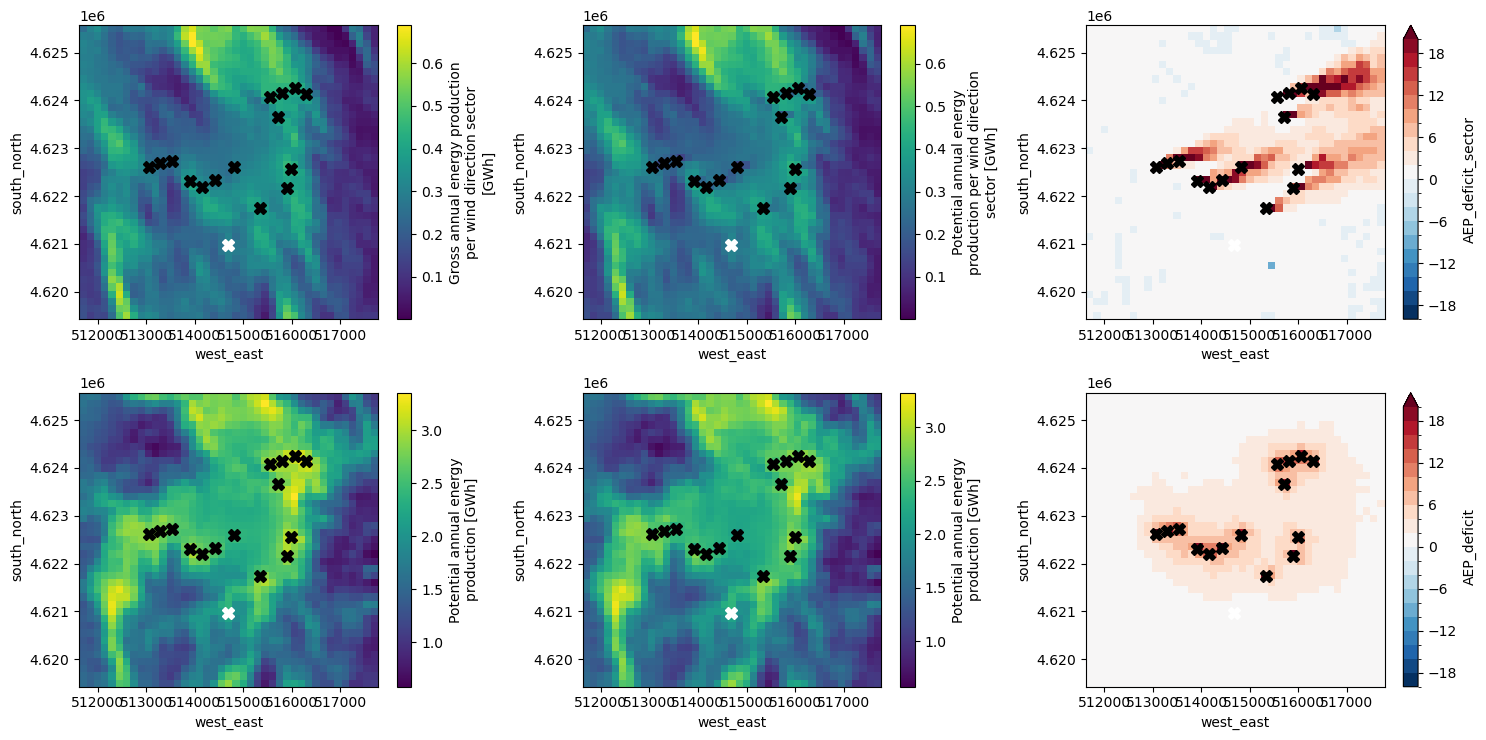
[ ]: